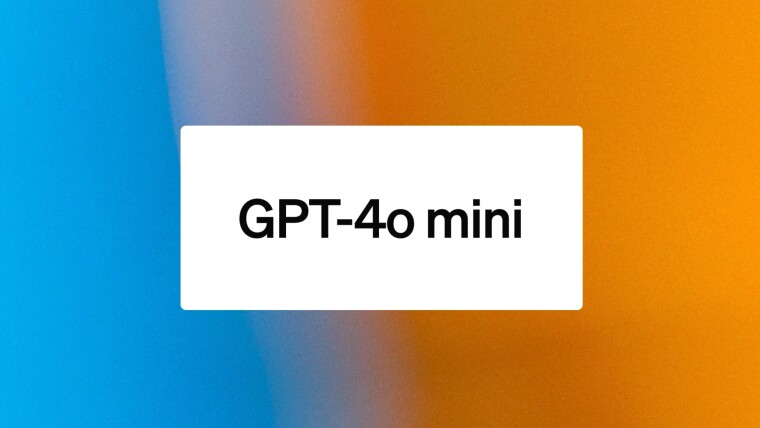
OpenAI says it has developed a new method to ensure large language models like GPT-4o mini are safe, without needing extensive human data collection, as was previously the case. The company said that this new Rule-Based Rewards (RBRs) method significantly enhances the safety of its AI systems.
The ChatGPT-maker revealed that it has been using RBRs as part of its safety stack since the launch of GPT-4, including its latest model GPT-4o mini, and it plans to use it in newer models in the future. RBRs use clear and simple step-by-step rules to check if outputs meet safety standards and avoid "the inefficiencies of recurrent human inputs" - this could help speed up the development time of future models.
Not only do RBRs use simple rules to train LLMs to answer safely, but they also reduce the number of incorrect refusals. Asking a language model an innocent question that it thinks is dangerous, perhaps because of the double meaning of a word, can be frustrating for users. LLMs trained with an RBR should still maintain high levels of safety without getting it wrong.
While a big step forward, RBRs do have some limitations. OpenAI said they"re best used for tasks with clear and straightforward rules. When it comes to more subjective tasks like writing a high-quality essay, RBRs struggle. Explaining how this issue can be addressed, OpenAI said:
"RBRs can be combined with human feedback to balance these challenges. For instance, RBRs can enforce specific guidelines (like "Don"t use slang" or rules in the Model Spec), while human feedback can help with more nuanced aspects (like overall coherence). The strength of the RBR is optimized to correctly enforce safety preferences but not impact the final reward score more than needed - in this way the RLHF reward model can still provide strong signal on e.g. writing style."
While OpenAI has highlighted the use of RBRs in making LLMs safer, the company did point out that RBRs are not limited to safety training. It said they can be used wherever explicit rules can define desired behaviors such as building a personality or format of model responses for specific applications.