Earlier this year, we explained how Google plans to use Federated Learning of Cohorts (FLoC) to prevent individual tracking while still serving you relevant ads. However, it announced that it is delaying this initiative just over a month ago, thanks in part to the massive backlash the company received.
That said, big tech organizations are still investing significant effort in privacy-preserving methods of data collection. Now, Facebook has revealed more details about how it plans to use privacy-enhancing technologies (PETs) to power the next generation of digital advertising.
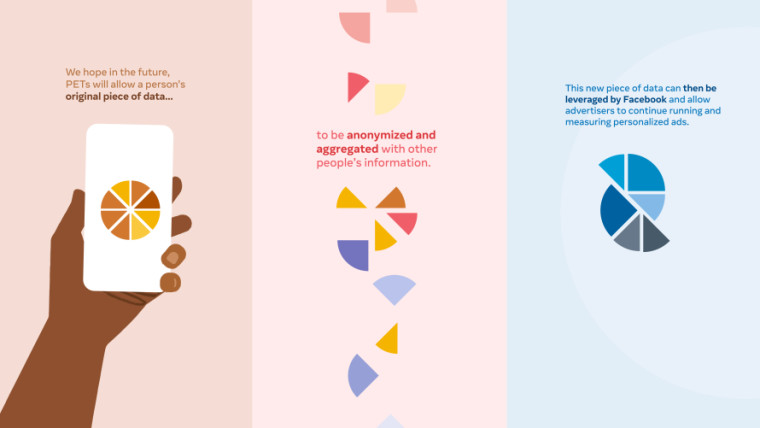
Facebook has stated that it is using techniques based on cryptography and statistics to implement PETs that allow it to reduce the amount of data processed while preserving your privacy, ad accuracy, and personalized preferences. The company has described three methods it is testing in its PETs effort.
The first is secure multi-party computation (MPC) which allows multiple organizations to process parts of your data and then share insights with each other. This essentially means that no single party holds all your data so possibilities of learning about you are reduced. An example of this is one organization holding information about what ads you are seeing and the other seeing information about what purchases you're making. MPC would ensure that both stakeholders would get the data they require without getting access to your entire data. Facebook is working on MPC using a solution called Private Lift Measurement based on its open source framework on GitHub. The company expects to make this solution available to advertisers next year.
Next up is on-device machine learning which ensures that algorithms learn from your data right on your device without sending the data to any external identity, the cloud, or a remote server. This technique is still under investigation and Facebook hopes that it will improve with time, if successful.
Finally, we have differential privacy, which is actually an add-on to existing PETs. The company describes it as:
Differential privacy works by including carefully calculated "noise" to a dataset. For example, if 118 people bought a product after clicking on an ad, a differentially private system would add or subtract a random amount from that number. So instead of 118, someone using that system would see a number like 120 or 114.
Adding that small random bit of incorrect information makes it harder to know who actually bought the product after clicking the ad, even if you have a lot of other data. As a result, this technology is often used with large data sets released for public research.
That said, Facebook has highlighted that these are all long-term efforts and it'll be sharing more information about its progress regularly.
6 Comments - Add comment