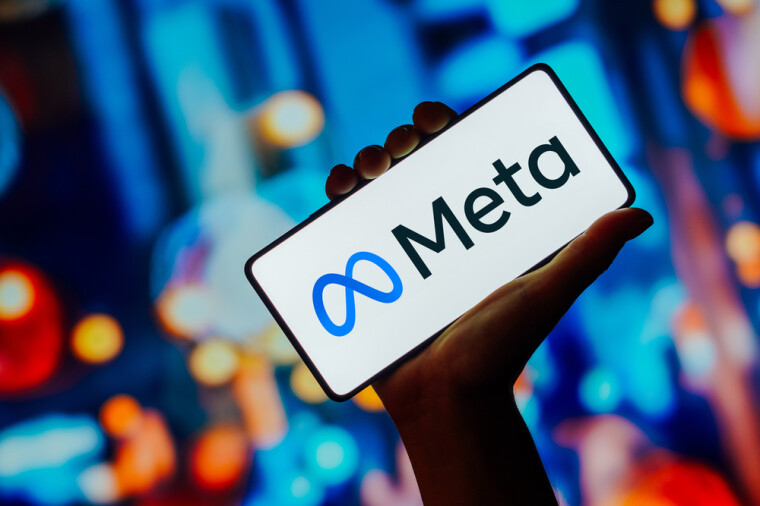
Segmentation is a crucial part of computer vision, used to identify which image pixels belong to an object. It finds applications in various real-world scenarios, from analyzing scientific imagery to editing photos. In 2023, Meta democratized segmentation by announcing the Segment Anything project, releasing both the Segment Anything Model (SAM) and the Segment Anything 1-Billion mask dataset (SA-1B) to accelerate research in this field.
Yesterday, Meta announced the Segment Anything Model 2 (SAM 2), which is more accurate and six times faster than the original SAM. Additionally, SAM 2 now supports object segmentation in both videos and images.
Key Highlights of the new SAM 2 model:
- SAM 2 significantly outperforms previous approaches on interactive video segmentation across 17 zero-shot video datasets and requires approximately three times fewer human interactions.
- SAM 2 outperforms SAM on its 23-dataset zero-shot benchmark suite, while being six times faster.
- SAM 2 excels at existing video object segmentation benchmarks (DAVIS, MOSE, LVOS, YouTube-VOS) compared to prior state-of-the-art models.
- Inference with SAM 2 feels real-time at approximately 44 frames per second.
- SAM 2 in the loop for video segmentation annotation is 8.4 times faster than manual per-frame annotation with SAM.
Since SAM 2 is available under the Apache 2.0 license, anyone can build their own experiences on top of the SAM 2 model. Meta is sharing the following artifacts:
- The SAM 2 code and weights under a permissive Apache 2.0 license.
- SAM 2 evaluation code under a BSD-3 license.
- The SA-V dataset, which includes ~51k real-world videos with more than 600k masklets, under a CC BY 4.0 license.
You can find the research paper on the SAM 2 model here and check out the web-based demo experience to see the model in action.
The potential applications for SAM 2 are vast, spanning across industries and research fields. By making the model available under an open license, Meta empowers developers and researchers to innovate and build upon this foundation.
Source: Meta - Image via Depositphotos.com
0 Comments - Add comment