Microsoft is heavily invested in artificial intelligence models with expertise in natural language understanding (NLU). To that end, the company has acquired startups studying natural language processing (NLP) and also has an exclusive license to OpenAI's GPT-3 language model. Now, the Redmond tech giant has announced that its AI model has outperformed humans in SuperGLUE benchmarks.
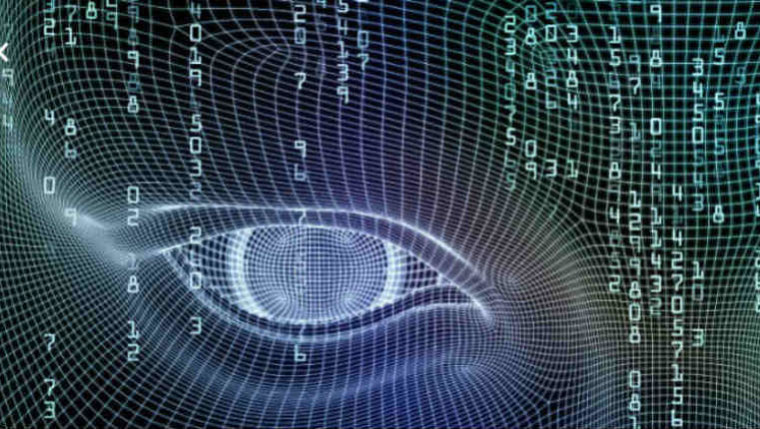
SuperGLUE is considered to be a difficult benchmark as it tests a variety of NLU operations such as answering questions when given a premise, natural language inference, and co-reference resolution, among many others. To tackle this benchmark, Microsoft updated its Decoding-enhanced BERT with Disentangled Attention (DeBERTa) model, and boosted it to have a total of 48 Transformer layers with 1.5 billion parameters.
As a result, the single DeBERTa model now scores 89.9 in SuperGLUE while the ensemble model with 3.2 billion parameters scores 90.3. Both of these scores are slightly higher than the human baseline of 89.8, which means that the model performs better than humans.
It is important to note that this is not the first model to surpass human baselines. The "T5 + Meena" model developed by the Google Brain team scored 90.2 just a couple of days ago, on January 5. However, Microsoft's DeBERTa even outperformed that model on January 6.
Moving forward, Microsoft has noted that it is integrating DeBERTa into the Microsoft Turing natural language representation model (Turing NLRv4), which means that it will then be utilized by customers across Bing, Office, Dynamics, and Azure Cognitive Services. The company says that the fact that its model uses fewer parameters than Google's solution means that it is more energy-efficient and is more maintainable because it is easier to compress and deploy. It went on to say that:
DeBERTa surpassing human performance on SuperGLUE marks an important milestone toward general AI. Despite its promising results on SuperGLUE, the model is by no means reaching the human-level intelligence of NLU. Humans are extremely good at leveraging the knowledge learned from different tasks to solve a new task with no or little task-specific demonstration. This is referred to as compositional generalization, the ability to generalize to novel compositions (new tasks) of familiar constituents (subtasks or basic problem-solving skills). Moving forward, it is worth exploring how to make DeBERTa incorporate compositional structures in a more explicit manner, which could allow combining neural and symbolic computation of natural language similar to what humans do.
Microsoft has released the model, its documentation, and its source code for public use on GitHub here.